The whole reason to have races and hackathons is to stress-test your code and find problems (ideally so you can fix them and do better next time). So the key part of any event is the post-mortem — understanding what didn’t work and why.
Here are a few of those from this week, both at our own DIYRobocars race in Oakland and from a ForumulaPi team.
Oakland DIYRobocars Event lessons:
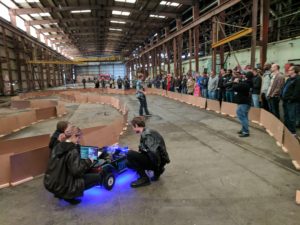
- 12 pizzas are not enough
- One bathroom is not enough
- We need a PA system
- The lighting in the warehouse ranged from bad to worse, which is really a problem for computer vision. At peak sun, the RGB lanes were read as RGB. But when the sun was obscured by clouds, gray became blue and contrast thresholds weren’t hit. Disaster. We can’t do much about the lighting, but competitors must use automatic gain adjustment algorithms in their code.
- We need to paint the lines on the concrete — tape and huge puddles don’t mix
- Otherwise it went great!
Keras/TensorFlow car lessons from Will Roscoe
[This describes the experience with his
“Donkey” Keras/TensorFlow CNN learning code] Overall today was a success given that two Donkey vehicles did at least one completely autonomous lap prior to race time. This is a big improvement from the same event 3 months ago when we worked on Adams car all day to get it to lurch forward and turn right. This is short debrief of what I learned this weekend to help guide our next efforts to make Donkey a better library for training autopilots for these races.
Of course, after the race the driving problems became obvious.
- The vehicle drive loop was only updating every 1/5th of a second but should have been updating every 1/15th of a second or more frequently. This meant we didn’t collect very much training data and the autopilot didn’t update often.
- Training data was not cleaned. We didn’t have a good way to view the training data on the ec2 instance so we could not see that there we were using training data that included bad steering, and even images when the vehicle had been picked up..
Beyond bugs, how can do better at these races?
On a race day, we have 4-6 hours to take a proven car and autopilot and train it to perform on a new track. The more efficiently we can improve the autopilot performance the better we’ll do. Here’s an overview problems I saw today and proposals how to fix them. Specific issues and solutions live in github issues.
Many steps are required to update an driving model.
At one point today I had 20+ terminal windows open because changing the autopilot requires many useless “plumbing” steps. These steps can be automated or made easier with command line arguments or web interface.
- Switching models requires restarting the server
- It’s difficult to remember which session was the good session.
- Combining sessions is a separate script.
Driving is required to test models.
Since updating and driving an autopilot takes time, we need to make sure that our changes actually improve the autopilot before we test it on the track. A trusted performance measurement is needed at the time of training. This could be a combination of the error on a validation dataset and a visual showing how closely predicted values were to actual.
There is no way to debug an autopilot.
Currently an autopilot either works or it doesn’t. Driving performance are the only clues to help us understand what’s going wrong. Helpful clues would include:
- Visual showing what the network is queuing off.
- Lag times
- Predicted vs actual overlaid on image.
Common problems that don’t have obvious solutions.
Even after a common problem has been identified, there’s no standard solution to fix the problem. “Agile training” could be used to correct the autopilot by creating more training data where the autopilot fails.
- Vehicle doesn’t turn sharp enough.
- Vehicle doesn’t turn at all on a corner.
- Vehicle goes to slow.
There is no easy way to clean training data on a remote instance.
Training on bad data makes bad autopilots. To learn where bad training data exists you need to see the image the recorded steering values, This is impossible on a CLI but would be possible through a web interface.
There are other improvements we can make but these are the big unsexy ones that will help most. Also, get a friend to build a Donkey. Let the fleet learning begin.
ForumulaPi car lessons from Jorge Lamb:
To train the machine learning we needed to generate many images and tell what we would want the car to do in each case. To do that we integrated the race code with the wiimote (cwiid) and played in the simulator: This way we stored the simulated camera images and the position of the wiimote as desired driving direction. It was even fun!
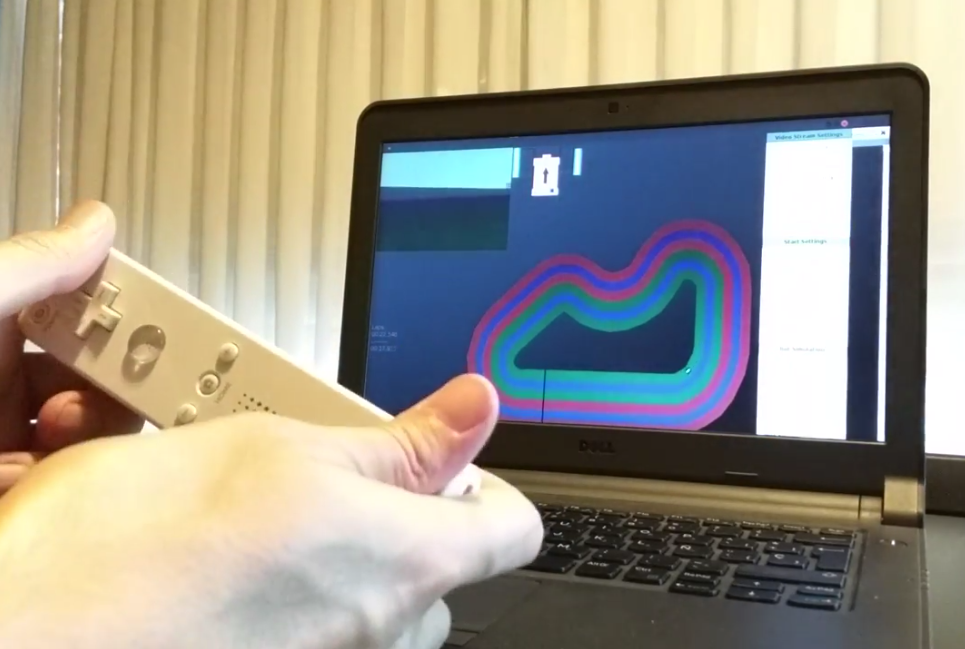
When we had enough images we started training a scikit-learn neural network. We started our tests with a fully connected neural network with one hidden layer. We did some changes to the wiimote driving code to train it to recover when it went out of the ideal path in the track. We run our code and the simulator in our laptop and it looked promising
We sent that for the first tests but some issues with the library, not compiled for the Raspberry Pi Zero made our robot stay in the starting line 
In the first race we had the library properly installed and so the neural network was going to drive the robot. This time the robot moved but our robot kept crashing against the walls, and in the recover movements it managed to do one full lap… in reverse! At least there was one other robot that did 5 laps in reverse :p
From the logs and images we got, our theory is that the Pi Zero took too long for processing each image with our code. This means it applied the driving directions to late and during too long. If it decided to turn, then turning for a full second meant driving into the walls.
For the next races we changed back to a simpler racing code: Follow the lane one right from the center.
In race 2 we did better (it’s easy to do it better than -1 laps
This time we managed to get nearly 10 laps for a second place in the heat, but that only gave us 1 point. First point though, yeah!
We kept testing and training neural networks, but we couldn’t get some of them to run in a Raspberry (after the issues in the test rounds we decided to test everything in a Raspberry Pi). Some libraries didn’t even run in the raspberry, and some other tests were too slow to properly drive the robot. Running some tests at lower robot speed, it looked really promising, but when driving full speed as you want to do in a race we didn’t find a working solution that didn’t crash into the walls.
We also tried convolutional neural networks. With neupy and a convolutional neural network that would fit in the Pi Zero it got many images right, but some others were giving wrong values and the robot would crash in the simulator.
In Race 3 we did even better! 16 laps and just a couple of meters away of house robot (which was surprisingly stopped). Our robot even passed the house robot a few seconds after the 15 minutes finished. 14 more points for RasPerras del Infierno 
Race 4 was even better: First win for RasPerras del Infierno!! 22 laps even though we were stuck with other robotfor a couple of minutes.
Race 5 start was very good but we crashed on a robot that went backwards for some time. Then there was a good competition and two other robots went over the 23 laps limit. A third place in the race, 11 points and one of the 10 places into the semifinal! (good luck the house robots are not going into the semifinal).